- Manto Team
How Actionability and Supervision fit into Employee Turnover Analytics Predictions
Updated: Feb 5
Over the last decade, employee turnover has skyrocketed from around 17% in 2010 to a potential 33% in 2020, nearly twice as high. 33% is a staggering number--one in three employees is predicted to change jobs over the course of the year. Not only are employee resignations increasing, the cost of each one is higher than ever. Companies are requiring more and more specialized and complex training for each new employee and more money is lost every time an employee leaves.
The data shows that the vast majority of employee turnover is preventable. Analytic predictions can identify employees who are likely to resign soon based on a complex series of data. While a traditional prediction may factor in things like age, company experience, relationship with management, and sick day use, next-generation solutions include criteria like professional training or even their commute time. Implementing these analytics allows companies to identify employees who are likely to leave and figure out how to meet their needs and keep them employed.
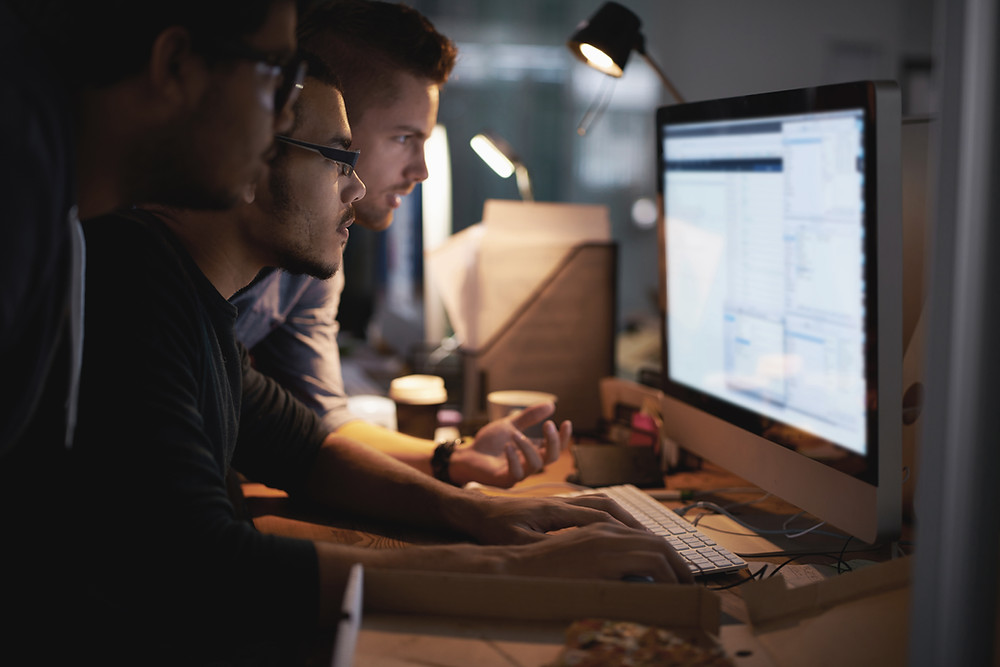
Actionability and Supervision
Analytics predictions are tremendously useful. However, there are two major features of turnover metrics that you need to keep in mind: actionability and supervision. Supervision also includes the ideas of ethics and diversity, but it all falls under the supervision umbrella.
Actionability
Simply knowing an employee is likely to resign soon doesn't do anything useful. Aside from giving them a bonus or extra time off, you're wildly guessing at how to solve their problem if you don't know why they're leaving. Actionability refers to what you can do with the data, specifically to encourage them to stay in their position. Any analytics system worth its salt will clearly provide the data that it drew a conclusion based on. Once you analyze the reasons the prediction system thinks an employee is going to leave soon, you can ask yourself two questions: "How can I encourage this employee to stay?" and more importantly, "Is this an issue that will affect (or has already affected) other employees? Actionability isn't just about why things happen, it's about what you can do to prevent it.
Supervision, Ethics, and Diversity
Along with actionability, it's important to understand (and be transparent about) supervision, ethics, and diversity.
Supervision: "supervision" refers to a human observing the analytics to ensure that the system generates data in a compliant way. This covers potential bias as well as allowing the human to sanity check the conclusions. A good analytic system will provide the logic behind its system, similar to a chess computer presenting possible lines.
Interpretability vs Explainability
Interpretability and explainability are two sides of the same coin, enabling actionability and supervision. Luckily, they're more straightforward to explain. Interpretability is simply breaking down the causes of the data-drawn conclusion. Since supervision requires a description of the causes that went into the conclusion, it requires on interpretability to provide them for review. Meanwhile, explainability attempts to explain those causes based on the employee's current record at the company. This then enables actionability. Just as you have to know where you've been to know where you're going, explainability provides the groundwork for actionability.
Let's take a look at an example: Manto flags an employee with three years of experience as likely to quit soon. The employee has used multiple sick days in the past month and their workload has decreased. Additionally, they've been late to work at least once a week for a couple months. Here's how actionability and supervision would fit into the program's decision-making.
Actionability: Instead of simply informing the user that the employee is likely to leave soon, Manto will focus on the signs and causes. If the employee fits the profile of a disillusioned worker who feels stuck in their position and doesn't care about their work, it may be prudent to schedule an evaluation or a 1-on-1 with a supervisor. Based on what Manto perceives the causes to be, it will provide feedback that management can use to help the employee feel valued within the company. Interpretability is simple in this case. Manto believes the employee is preparing to quit because of absences, tardiness, and decreased workload.
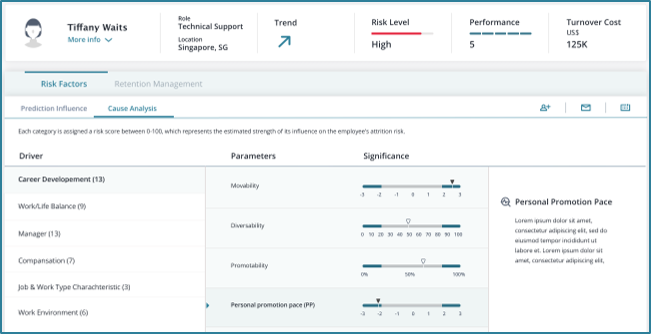
Supervision (plus ethics and diversity): Because Manto provides a detailed description of why it drew the conclusion that the employee was considering resigning, a human can review the data. Perhaps the absences fell on religious holidays or there was a death in the employee's family. As for the decreased workload, a human supervising the program could verify other extenuating circumstances. It's entirely possible that the employee is planning to leave shortly, and Manto will flag that with a high degree of probability. But supervision will make sure you're getting the whole story. On the explainability side: Absence and tardiness are certainly signs of an employee on their way out, but they're symptoms and not causes. In explainability, Manto provides the relevant data and assists human supervisors in figuring out why the employee is leaving. If the root cause can be addressed, perhaps the issue can be resolved and the employee will return to their usual productive self.
The Manto Bottom Line
The price of employee turnover is massive - Almost every empirical study shows that an employee resigning can cost her employer anywhere between 30% and 150% of the employee's annual salary. That means a company with 2000 employees, 15% turnover rate and an average annual salary of $100K will most likely lose 20-30 million dollars each year on employee-turnover related costs.
Additionally, over three-fourths of employee resignations are likely preventable. Even a small investment in keeping employees satisfied and working can pay major dividends. The issue with current attempts to increase employee retention is simple: they don't start until after the employee's decision to leave. In the rare case that the company can change the employee's mind, they're missing the chance to reach out and fix the issues before they overwhelm the employee. Proactively analyzing employee behavior will allow you to predict when they're planning to leave, but it will (more importantly) allow you to make their day-to-day work more satisfying.
Employee behavior prediction is attractive because of the potential to save millions of dollars in turnover costs. But the true value goes beyond that. For whatever reason, employees are often hesitant to raise fixable issues with management. With the ability to proactively fix them, management can solidify bonds and increase job satisfaction with all their employees.